Type | |
Stats | 431 415 1.8k |
Reviews | (120) |
Published | Dec 2, 2024 |
Base Model | |
Training | Steps: 1,380 Epochs: 23 |
Usage Tips | Strength: 1 |
Hash | AutoV2 2366A18968 |


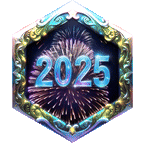
Flux Lora for Playing Card Images
Trigger Word for v0.2: pcardimage
v0.3 dose not require a trigger word, you can simply use phrases like playing card image, or other phrases to invoke the playing card style. experimentation may be required.
v0.3 Update notes:
v0.3 has been trained on fewer images prioritising quality over quantity, has been trained using captions instead of tags, has been trained on a lower network dim allowing for easier change of styles via prompt and hopefully smoother blending with other style loras. This is still not perfect and I still want to try with a more diverse dataset but is a massive improvement to v0.2.
I began learning how to create LoRA models with the goal of designing my own AI-generated deck of playing cards. This is my second attempt at creating this LoRA file. My first attempt was a complete failure, so I shifted focus to simpler styles to better understand how to train models effectively. This version, while still not perfect, is a significant improvement and is usable. However, I plan to continue fine-tuning and experimenting further. This page serves as a testing ground for storing my results and tracking my progress.
The model is not specifically trained to create entire playing cards; rather, it is designed to generate the artwork for the cards, particularly the mirrored, flipped imagery seen on picture cards. It was trained on a dataset comprising scans of four decks of playing cards. The scans were manually edited to remove pips and numbers to focus on the artwork.
Current Limitations
Overly Strong Model Influence: This version tends to default to imagery closely resembling the training dataset, limiting its ability to generate diverse characters or faces. Lowering the model strength can mitigate this issue, but it still requires significant trial and error to achieve the desired results.
Style Limitations: The model heavily reflects the styles present in the training dataset, which include monochrome, traditional, faded water-damaged, and abstract art styles. While this aligns with the training data, it may not always be ideal for certain creative goals.
Planned Improvements
My next steps include expanding the dataset to include a broader variety of styles. I plan to train the model on a larger dataset with one or two images each from approximately 50 different decks. This diversification should help reduce the model's reliance on specific styles while improving its ability to generate diverse, mirrored imagery without directly copying the training data.
Caution for Users
If you use this model on platforms like Civitai, please note that it may produce many unusable results due to its current limitations. Experimentation with parameters and model strength is often necessary to achieve satisfactory outputs.
Feedback and Suggestions
I welcome any feedback, suggestions, or assistance in refining this model. This is a project I am passionate about, but I’m still learning how to teach the model to create the mirrored imagery characteristic of playing cards without overly replicating the specific style of the training dataset.